Early fire detection system
A fire is considered large when it exceeds 500 ravaged hectares. If we review the statistics of recent years, we see that, with each passing year, the number of large fires continues to increase. In 2019, for example, there were 15; in 2020 the figure was increased to 18; in 2021, the figure reached 21; and in 2022, up to October, we’ve already had more than 60.
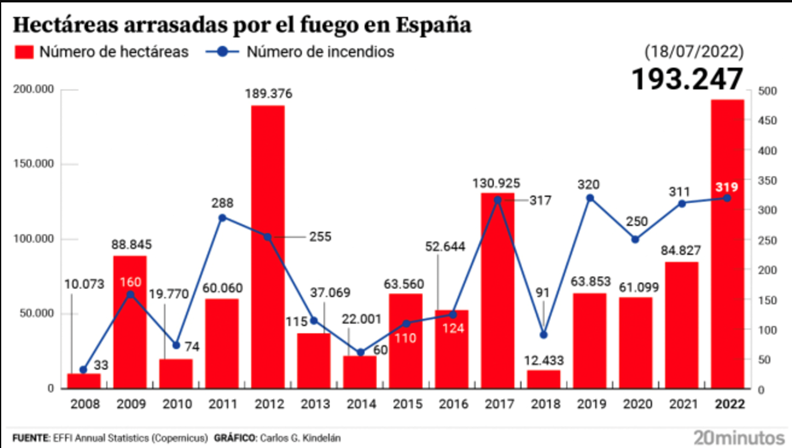
It is a clearly growing problem, and both prevention and quick action measures must be taken to minimize the number of these large fires, since fires not only cause the loss of forest hectares, but are also associated with several consequences, many of them irreversible.
Depending on the devastated natural areas, recovery can take between 5 and 200 years, not to mention the elimination of complete ecosystems that in many cases cannot be recovered. In addition, the destruction of public and private properties directly affect the economy of the territories and the primary sector such as livestock and agriculture, as well as the direct risk it poses to people.
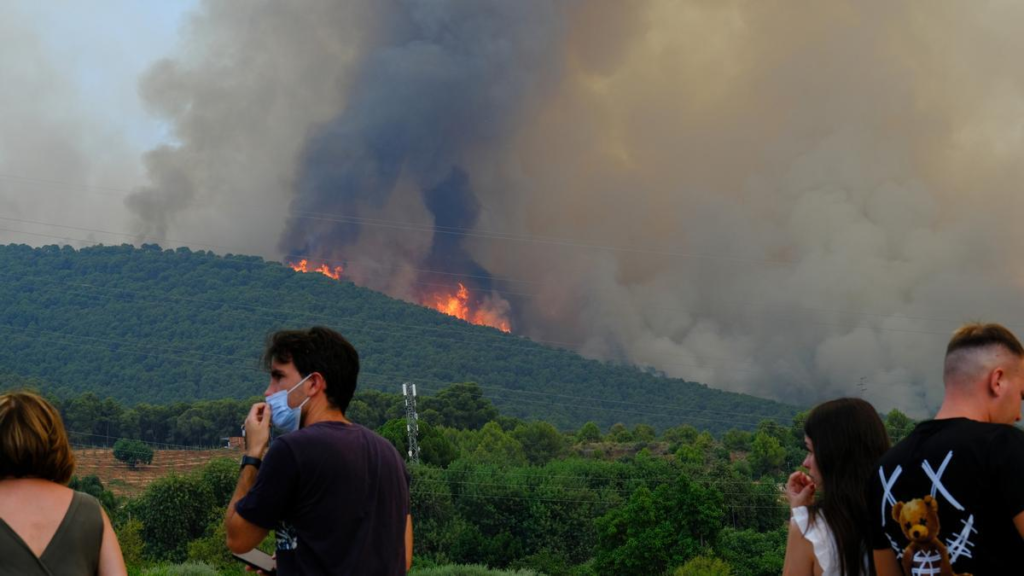
Considering the consequences of each fire, we must focus on the problem and seek current solutions that help us prevent these fires. To do this, we at Minsait are developing an early fire detection system, aiming to generate alarms for rapid action of reserves, thus improving the protection of flora, fauna, infrastructure and human lives.
To solve the problem, we have designed a completely autonomous system for its deployment in the open field, with the necessary capacities for the study of the environment, by capturing information from the environmen, through sensors and cameras, thus enabling in-time processing, and for the sending of the alarms that are pertinent to a fire detection.
Detection system operation
The basis of operation is the use of artificial vision to detect and regonize images. The image processing system is carried out by deploying an AI model trained to detect smoke columns. From an image, the system will analyze and identify whether there is a fire or not.
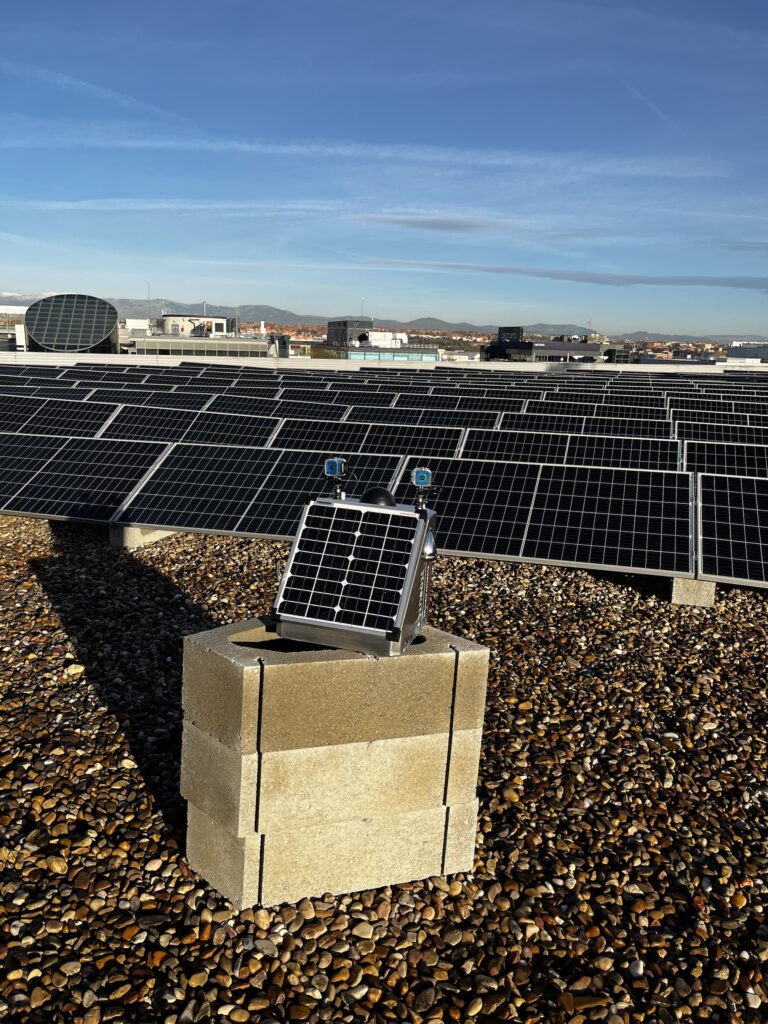
We will next see a simulation of a scenario in which our model would activate:
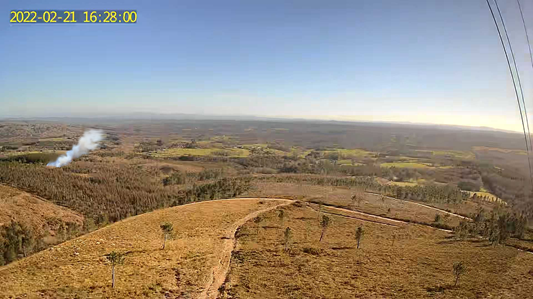
At a functional level, we see, with a range of probability, that what is detected in the image is an outbreak of fire. This is what it would look like in the tests of the training that we carried out:
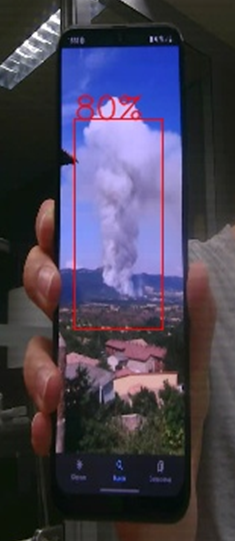
As we can see, the algorithm is capable of detecting column-shaped structures of smoke so as to anticipate a much larger fire.
Continuing with the images, one of the main problems when carrying out the study of the images, was the noise generated by the environment, where we found clouds, reflections of the sun, etc., all of them causing false positives that had to be solved. To do this, we apply a mask to rule out the sky and urban centers. In this way, we veto false positives of flashes coming from the sky or from population centers.
Components
The device has the following systems to work autonomously:
Autonomous supply system
There is an autonomous power and charging system in the equipment, made of a front solar panel for the electrical supply, a charge regulator to control the battery charge state, so as to guarantee that optimal filling is carried out, and the battery responsible for powering the system.
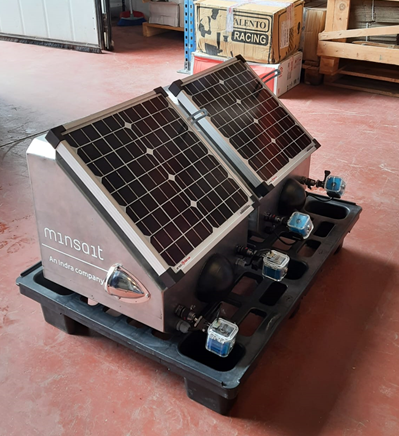
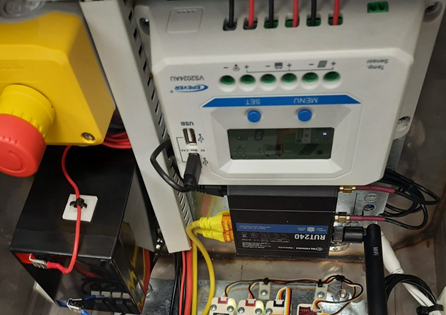
Surveillance system
It is the system in charge of capturing the images necessary for processing in real time, capturing the environment in 360º thanks to a system of several cameras. Additionally, the device has a PTZ camera for maintenance tasks, visual validation in the event of an alarm, and remote surveillance.
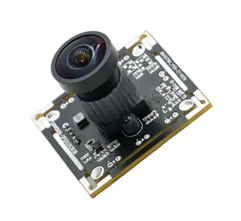
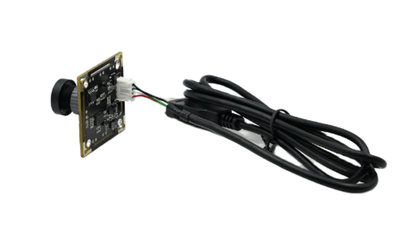
Environment system
Several sensors are available to support fire detection, since weather conditions must be taken into account at all times. Thanks to the sensors, we control the start of the modem and of the inference system.
Processing system
The equipment has an IoT Gateway with capacities for the acquisition and storage of information, thus allowing real-time processing and early alarm detection:
- Alarm system: the IoT Gateway itself has capabilities to create flows for custom-made development, thus being able to define the behavior upon detection of an alarm.
- Inference engine (RBP): it is in charge of processing the images and sending the results obtained. Image processing consists of 4 phases:
- Image taking.
- On the taken images, making the inference (either there is fire or there is no fire).
- Detection of people and vehicles, with the possibility of identifying license plates or suspicious behavior.
- Masks: if the result of the inference is positive, applying these masks allows us to rule out false positives, since the masks omit the sky and urban centers from the image, thus eliminating possible flashes or lights that could have confused our model. In this way, we will keep the positives that persist once the masks have been applied.
- Uploading the results to a server to be viewed.
Communication system
The device has a modem and 3G/4G connection to send information to the cloud, thus allowing outbound exit and remote communication of the device.
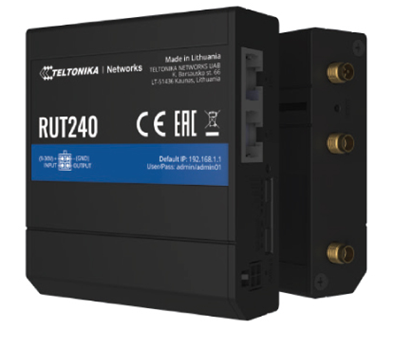
Device management
As we have mentioned, the device is powered by a solar panel that recharges the battery which in turn allows us to power all the systems, so the control of the battery must be very accurate to avoid consuming more than necessary and to avoid completely draining the battery.
To do this, the device works through on- and off-cycles that are calculated based on the battery percentage.
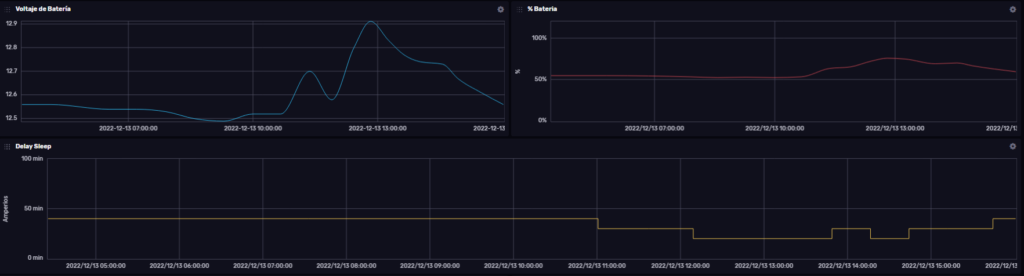
Specifically, in the green and red band, we can see that, for a given battery percentage, there is an associated time in which the machine remains off until the next cycle comes. That is to say: If when starting the device, the battery is detected to have a percentage of 60%, that means that, once the device is turned off, it will wait 25 minutes until it turns on again. In this way, we can make sure that the battery recharge is enough, thus the device will remain operational.
What do we do in an on-cycle?
When the device starts, it turns on the different devices that are inside it. Depending on the values taken, we will perform image taking and inference making.
For example, there are cases where we would not take any action when starting, such as when the humidity levels are very high and the atmospheric pressure is low, since we can assume that it is raining at that moment and, thus, we will consider that it is not a scenario in which a fire can happen. The system would turn off until it starts up again in its next cycle, and then recheck the sensor values.
To understand betterwhat the system does in a cycle, let’s have a look at the following diagram:
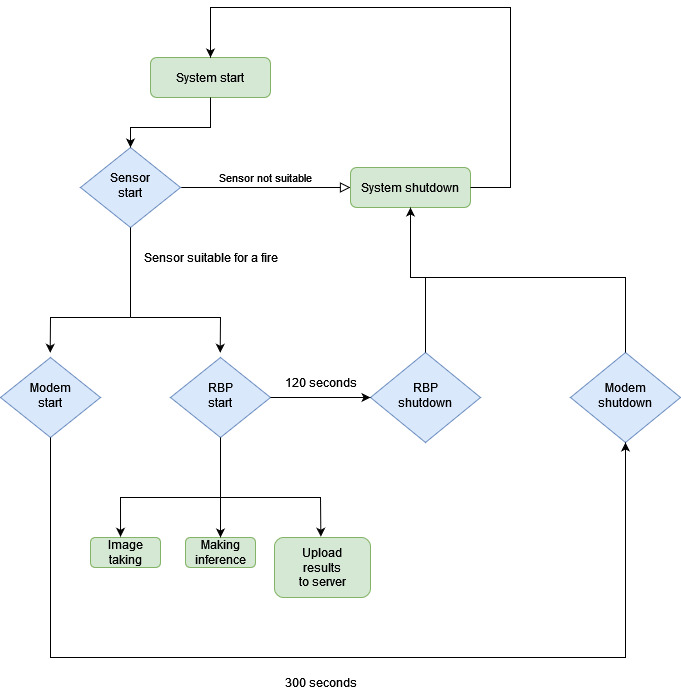
Due to what we have previously mentioned about the operation of the device, the first thing we receive are the values from the environment, then depending on the data obtained we turn on the modem and the inference engine. For this case, we use a Raspberry Pi.
The modem turns on and establishes a connection with the cloud, sending the values from the different sensors in JSON-formatted message through MQTT, to the environment associated with the device.
An example of one such JSON packets would be:
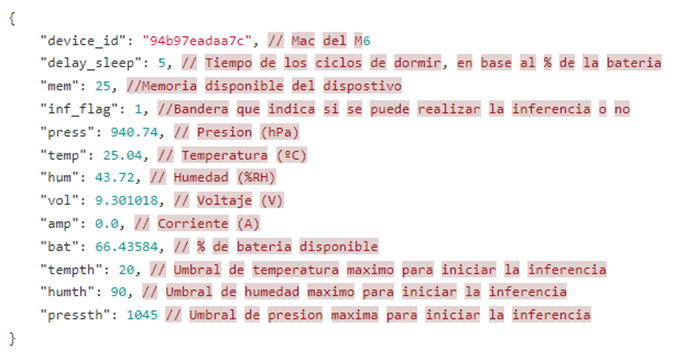
At the same time, the modem starts the Raspberry Pi, which has been configured to execute the following steps consecutively when starting:
- Image taking: obtaining the images of the environment.
- Uploading files to FTP: grouping the captured images.
- Making the inference and filtering masks: processing the images, detecting the possibility of fire.
Another point to highlight about the operation of the device is that we can send it commands:
- Maintenance command: this command allows us to have the device up, turning on only the modem and the Raspberry Pi to be able to connect remotely and perform maintenance tasks within the Raspberry.
- PTZ command: it allows an operator to access the PTZ camera to see the situation generated, in the event of a possible fire.
Current situation
At this time, the device is already deployed in the field, obtaining the following images of the landscape it monitors:
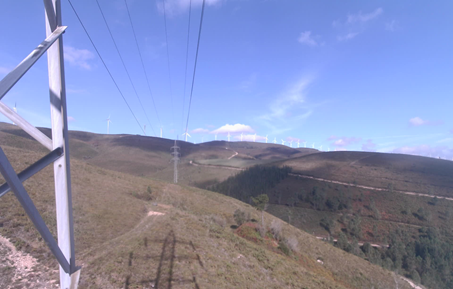
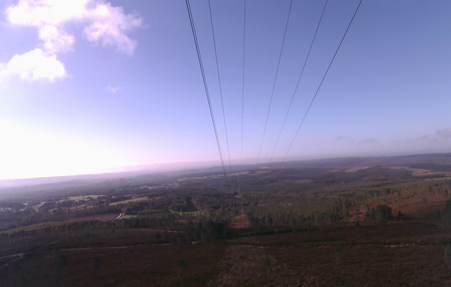
This device is currently in final tests, as it is part of an Innovation project in which we are working. With everything learned, the final version is being developed, which will start working very soon.
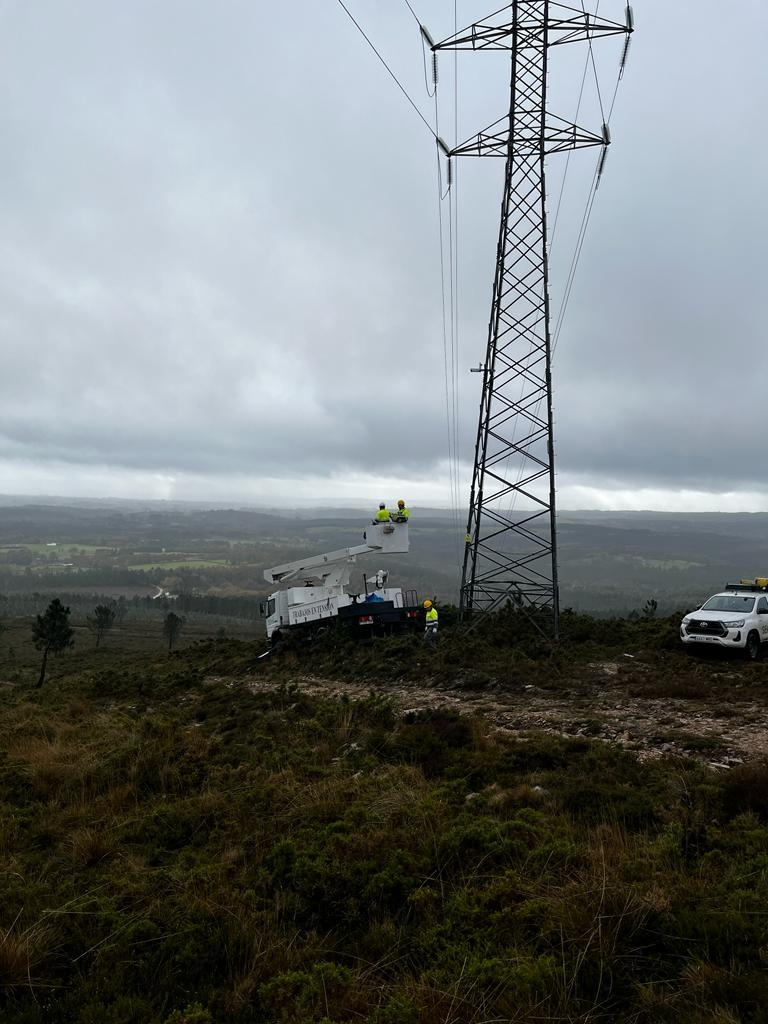
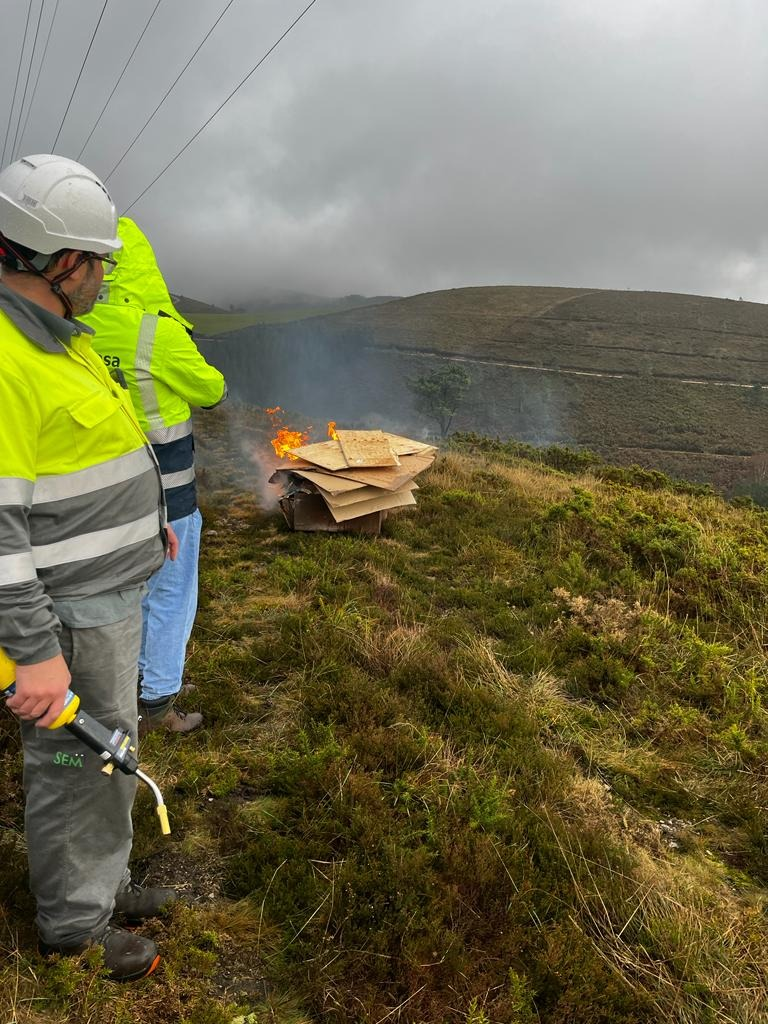
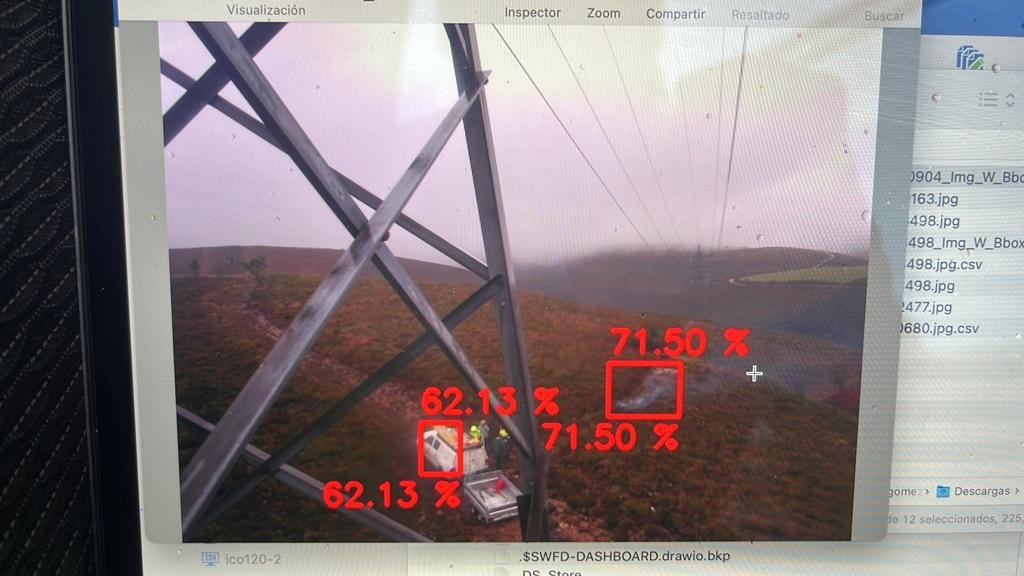
Header image: Malachi Brooks at Unsplash