Data Fabric: concept, capabilities and state of the art
It is not easy to define the concept of Data Fabric because the definition changes as the concept evolves from being initially associated with a product to an architectural and design approach to data management and integration. In fact, many vendors have created their own Data Fabric definition adapting it to their offering, creating even more confusion.
Currently, the interest around the concept of Data Fabric is very high, as there is a real need to better manage data for reasons such as:
- The shortage of qualified data engineers, making automation a necessity.
- The adoption of cloud solutions has introduced many special-purpose data warehouses for capturing and managing data.
- The increase in the number of data sources needed to be integrated and their diversity (structured, semi-structured and unstructured).
- Creating more data silos.
- Businesses face intrusion from events and external forces that require rapid analysis in the data environment. This drives the need to be able to integrate new data sources in an agile way.
- The need to reduce costs in the long term due to increased competition.
These and other factors drive the need for a Data Fabric, as it can help us automate and optimize data, allowing better data management and integration, while providing a uniform layer that can be accessed by all consumers of data.
Capabilities of a Data Fabric
To facilitate analysis, we are going to group the expected capabilities of a Data Fabric into these categories:
- Expanded Data Catalog : Being able to find, connect, catalog, and integrate all types of data and metadata is one of the core capabilities of a Data Fabric. The data catalog connects to metadata from all sources and automates some of the tasks related to using a data catalog, such as metadata discovery and ingestion.
- Knowledge graph enriched with semantics: This graph is designed and built to store and visualize the complex relationship between multiple entities. In addition, the taxonomies and ontologies used in the knowledge graphs must be intuitive to interpret and maintain for non-technical resources.
- Metadata activation: Traditionally, only passive metadata that is generated at the time individual data objects are created (for example, data type, length, and description) has been considered. Additional technical metadata such as transaction logs, user logs, and query optimization plans are considered in a Data Fabric. In combination with the knowledge graph, technical metadata is used to create what is called active metadata. The active metadata will be used as input to the recommendation engine.
- Recommendation engine: Based on active metadata, artificial intelligence (AI) and machine learning (ML) routines are applied to recommend data integration and delivery optimizations. For example, the recommendation engine may propose to convert a data stream implemented through virtualization to a near-real-time mode of delivery due to performance degradation.
- Data preparation and delivery: A Data Fabric enables self-service data preparation, allowing users to access the common semantic layer, represented by the knowledge graph, in an environment where they can explore and transform data to create new data sets. The second element, data delivery, is the ability to support different styles of data delivery to data consumers (for example: support for streaming, bulk / batch, and virtualization).
- Orchestration and DataOps: The orchestration layer will support better synchronization of data flows. Using DataOps principles throughout the process enables agile and repeatable data delivery.
These categories or pillars of a Data Fabric constitute a Data Fabric architecture designed to support the entire spectrum of data management and processing.
State of the art
As we have said, today the concept of Data Fabric is defined more as an emerging data management and integration design pattern than as a product. Implementing a Data Fabric requires a combination of different data management technologies, both known and emerging.
According to the Hype Cycle for Data Management (2021), the Data Fabric has an estimated market penetration of between 1% and 5% and is at the top of the Peak of Inflated Expectations. It has a horizon of five to ten years to reach full maturity.
In addition, some of the components of a Data Fabric are even less mature and therefore also have an even lower market penetration. For example, DataOps is still defined as an innovation driver with less than 1% market penetration.
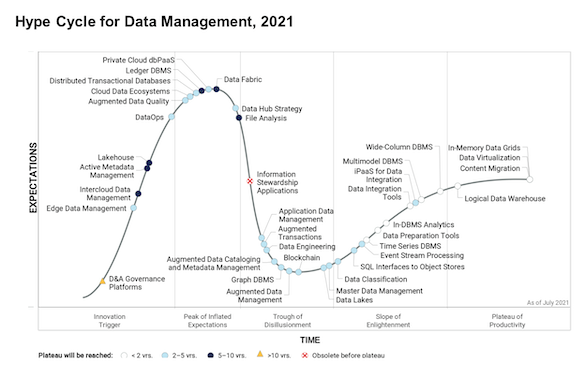
Currently, no vendor is able to provide complete coverage of all the pillars of a Data Fabric on a single, fully integrated platform, this means that it is not possible to simply buy a Data Fabric product that covers all capabilities.
What do you think? Do you think that this can be accelerated by the interest generated, or will we have to wait those five to ten years that are discussed?
Header photo by 🇸🇮 Janko Ferlič on Unsplash