Let’s talk Ops: DevOps, DataOps, MLOps & AIOps
Those of us who work in the TI world have become accustomed to hearing in recent years various terms that include the word ‘Ops’. This term refers to operations, and more specifically to the automation of operations with the aim of improving the service provided.
Perhaps where it is most commonly heard is in the integration implementation (CI) and continuous deployment (CD) of applications; the so-called DevOps. However, we also find it related to data management, machine learning and even artificial intelligence.
In this post we are going to review a little of what each of these automations consists of.
About DevOps
According to Microsoft, the term DevOps is a combination of the terms ‘development’ and ‘operations’ that designates the bringing together of people, processes and technology to deliver value to customers on an ongoing basis.
DevOps therefore allows previously siloed roles – development, IT operations, quality engineering and security – to coordinate and collaborate together to produce better, more reliable products. Teams thus gain the ability to be more responsive to customer needs, increase confidence in the applications they build, and achieve business goals in less time.
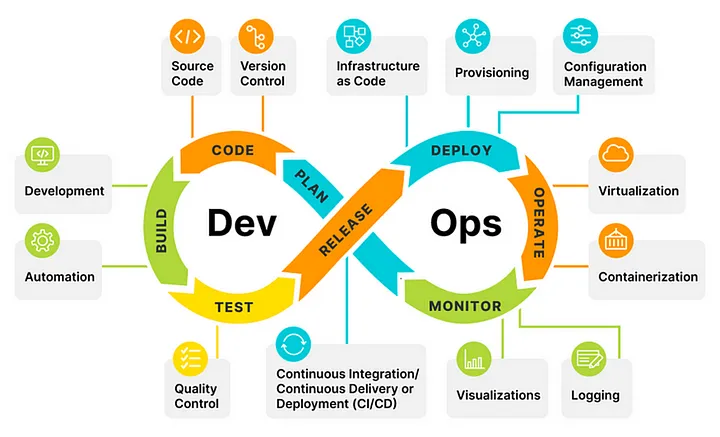
The benefits of DevOps are clear; teams that embrace DevOps culture, practices and tools improve performance and create higher quality products while using less time, resulting in higher end-customer satisfaction. This improved collaboration and productivity is also vital to achieving business goals.
About DataOps
DataOps is a collaborative data management practice that automates the delivery of data with an appropriate level of security and quality. This type of operation helps to generate high quality collaborative channels at scale for a large number of use cases in an enterprise or agency.
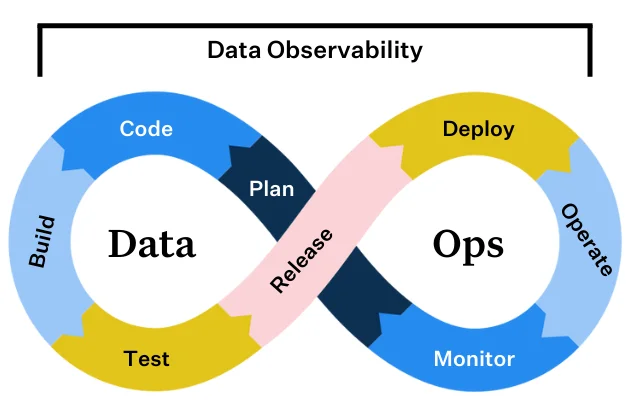
DataOps is based on the DevOps philosophy, but with a focus on data analysis with a quest for automation, speed and accuracy in the processing of such data. The advantages of this type of operations are:
- Avoiding duplication of data: as the aim is to create more accessible, quality and available data products.
- Creation of a data strategy: facilitating the collaboration of teams in all phases of development, so that data is available as soon as possible.
- Improved data analytics: thanks to the use of machine learning algorithms, being able to manage large amounts of data.
- Improved operational efficiency: efficiency, agility, security and data transformation change are optimised.
- Advanced processes: by adopting DataOps, enterprises and organisations accelerate the transition to the cloud and the execution of digital transformation strategies.
- Supporting automation technologies: eliminates the time required to execute manual tasks.
About MLOps
MLOps has emerged as a new trend, completely necessary in an AIML ecosystem, which has the main objective of maximising the performance of our ML models, increasing agility in model development and improving ROI.
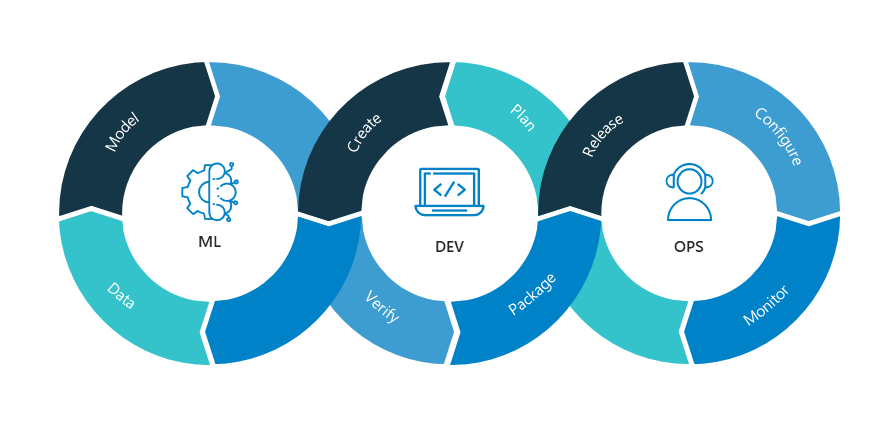
The goal of MLOps is to support the AIML ecosystem to manage the model lifecycle through automation, producing consistently reliable and quality results without performance degradation, and ensuring scalability of AIML products.
However, as expected, achieving this is far from easy, so a complete automated MLOps solution has several components including:
- Automated ML model building pipelines: Similar to the concept of continuous integration and continuous delivery (CI/CD) in DevOps, ML pipelines are established to build, update and make models ready to go into production accurately and seamlessly.
- Model Servicing: This is one of the most critical components, providing a way to deploy models in a scalable and efficient manner so that model users can continue to use the results of the ML model without loss of service.
- Model Version Control: This is an important step in an AIML workflow, enabling code change tracking, data history maintenance and cross-team collaboration. This provides scalability to give agility to experiments and reproduce experimentation results whenever necessary.
- Model Monitoring: helps measure key performance indicators (KPIs) related to the health of the ML model and data quality.
- Security and governance: This is a very important step to ensure access controls to ML model results and to track activities to minimise the risk associated with consumption of results by unintended audience and bad actors. Today, data is the real asset, so it is essential that this data is only accessible by the intended audience.
About AIOps
IBM defines AIOps as the application of artificial intelligence (AI) capabilities, such as natural language processing and machine learning models, to automate and streamline operational workflows. Through Big Data, analytics and machine learning capabilities, this type of operations will enable:
- Collect and aggregate huge volumes of data: generated by multiple components of the IT infrastructure.
- Intelligently separate the important: to identify significant events and patterns related to application performance and availability issues.
- Diagnose causes: and communicate them to IT and DevOps departments for rapid response and correction, or to automatically resolve problems without human intervention.
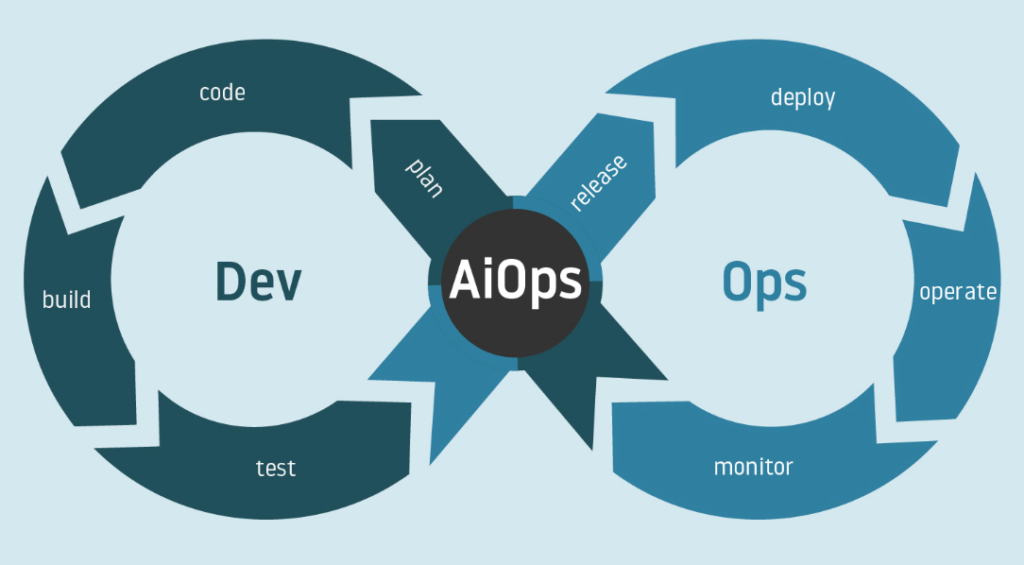
The benefits of AIOps can be summarised as follows:
- Faster mean time to repair (MTTR): by reducing IT operations noise and correlating operations data from multiple environments, AIOps can identify problem causes and propose solutions faster and more accurately than humanly possible.
- Lower operational costs: Automatic identification of operational problems and automatic response scripts will reduce associated costs.
- More observability and better collaboration: more effective cross-team collaboration is facilitated across DevOps, ITOps, governance and security functions.
In short
From what we have seen, managing a system from a single computer is at the end of its useful life as business processes become increasingly complex and IT systems continually change with new technologies. Implementation in detail involves a combination of collaborative practices, operations automation, monitoring and a focus on continuous improvement as part of DevOps, DataOps, MLOps and AIOps processes.
DevOps focuses primarily on IT processes and software development, and the DataOps and MLOps approaches focus on improving collaboration between IT and businesses and agencies, as well as the overall use of data in organisations. DataOps workflows leverage DevOps principles to manage data workflows. In the case of MLOps, it also leverages DevOps principles to manage embedded machine learning applications.
Header Image: Tingey Injury Law Firm at Unsplash.